An automated grape yield estimation system
Baden Parr & Dr Mathew Legg – Massey University, Auckland
Accurate yield estimation is essential for optimal vineyard and winery management.
Vineyards often have contractual requirements to wineries to produce a predetermined yield and quality of fruit. Vineyard yields often fluctuate year to year and wineries may not purchase more crop than required. Accurate yield estimations allow vineyard managers to plan for the coming season, such as organising vats, barrels, and labour. A vineyard’s location, its soil makeup, and climate are all factors impacting overall quality and yield. If a vine is carrying more fruit than optimal, the quality of the fruit at harvest will be negatively impacted. Also, understanding vineyard variability at a per-vine level can potentially facilitate the use of precision viticulture techniques such as variable rate application and selective harvesting.
Historically, yield estimation has been achieved several ways. Traditional approaches predict a season’s yield based on historical results and weather conditions. More modern approaches may combine historical data with periodic visual inspections that measure expected cluster counts and berry sizes. This approach can provide robustness to seasonal variations, however, is labour intensive, time consuming, and prone to human error. For best results, these manual inspections need to be carried out regularly from bloom to harvest.
An automated process for yield estimation is desirable as it could remove human error and increase the available temporal and spatial frequency of estimates. There are several promising techniques to achieving this. Laser scanners have been used to generate high resolution digital representations of vine canopies as well as individual bunches. Yet the equipment cost and sheer volume of data that needs to be processed somewhat limits its usefulness in real environments. Computer vision techniques have also been investigated. These use variations in colour and luminous intensity to locate and count grapes within high resolution colour images. These approaches face several practical challenges including limited colour contrast between grapes and the surrounding foliage along with variations in lighting due to unpredictable shading from leaves. Determination of 3D information provides additional information about the scene. Simple features such as an objects shape give information as to what that object may be without reliance on texture or colour cues. A 3D solution may lead to a more robust solution for grape yield detection in a wider range of situations.
NZ Wines has provided the Rod Bonfiglioli PhD Scholarship to fund a project that aims to develop a novel automated grape yield estimation system using low cost 3D camera technologies. Research will be conducted closely alongside the New Zealand wine industry to understand and address their needs with a focus on practical solutions for a future commercial output.
Investigated Depth Cameras
In recent years, new technology advances have led to the growth of low cost 3D camera technologies; including Stereographic, Structured Light, and Time of Flight (ToF). Each of these camera technologies have unique performance characteristics and trade-offs. An initial focus of this work is to understand how these may be used for grape yield estimation.
Stereographic cameras use a similar principle to human binocular vision, identifying the difference between similar points in two separate camera images and calculate the depth information using trigonometric principles. This can be achieved using generic pattern matching, or by specifically looking for known features within each image, such as grapes. With strong feature detection algorithms, this technique can be relatively accurate. However, it can struggle in scenes with limited detail.
Structured Light cameras project a known pattern of infrared light into the scene. The unique distortion caused by it falling on objects within the scene is captured by either a single or stereo pair of infrared cameras. Structured light cameras perform well in scenes with uniform smooth surfaces where the distortion pattern is relatively continuous. Unlike stereo cameras, the projection allows them the benefit of working in scenes that have otherwise undistinctive features. However, reliance on the projection of light means that quality can suffer in the presence of strong ambient light such as direct sunlight. Recently hybrid Stereographic & Structured light systems have been developed that combine the best of both technologies.
Time of flight cameras have traditionally been one of the most expensive 3D vision techniques. Instead of relying on projection or stereo principles, these approaches consist of a single camera paired with an infrared flash. By measuring the precise time it takes for light to return to the camera sensor, the depth information within a scene can be established. The miniscule time scale involved is the main contributor to the traditionally substantial price. However, recent advances in signal processing and integrated circuit fabrication have allowed for low-cost options to become available.
Evaluation setup
Six different depth cameras have been acquired and initial tests performed. Each camera has been tested within an office environment focused on two bunches of table grapes. The scene presented to each camera is shown in Figure. 1. Green and red bunches were chosen to investigate the impact colour may have on the results.
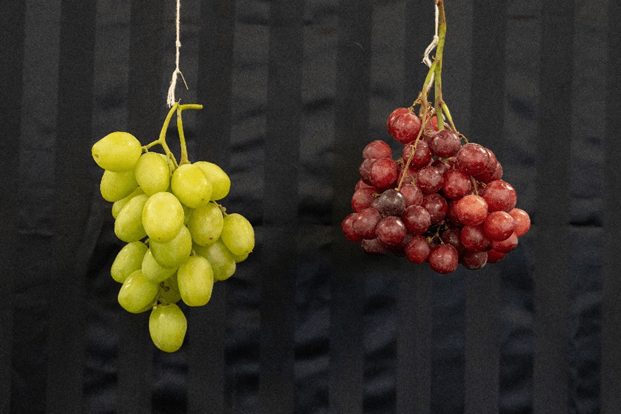
Figure 1: Table grapes used for initial testing of depth cameras.
While not indicative of a realistic vineyard environment, certain similarities remain. Table grapes have been used for convenience, providing the ability to perform quick measurements without needing to visit a vineyard. The grapes are an adequate proxy in shape and colour.
Initial Observations
Across all cameras tested, individual grapes were able to be distinguished. Structured light and Stereograpic cameras featured a slightly noisier image and overall smoother definition between individual grapes. In comparison, ToF cameras were able to resolve more fine detail. An interesting observation of the ToF camera was the pressence of strong reflective components appearing in the final depth image; seen as peaks on individual grapes. These will be investigated further as potenial features for novel machine learning techniques. Aditionally, signal processing techniques will be used to improve overall quality and reduce the effect of noise for all tested cameras.
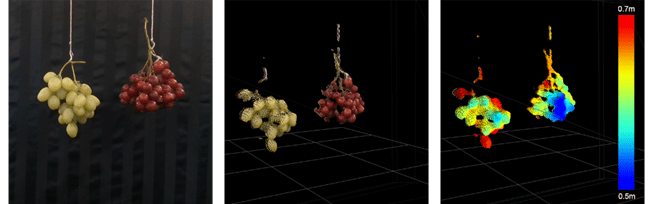
Figure 2: An example of a colour and 3D scan captured from a structured light camera. Colour image (left), coloured point cloud (middle), and point cloud depth map (right).
It is worth noting that these tests were conducted with “out of the box” configurations. There are a number of approaches that are expected to significantly improve accuracy and reduce noise.
This article first appeared in the December/ January 2018 issue of the New Zealand Winegrower magazine.