Martin D – Plant & Food Research: Marlborough
Overview
The basic idea that is being tested within the Bragato Research Institute (BRI) Pinot Noir Programme is that the apparent link between wine quality and vine productivity can be broken. To break this quality/productivity seesaw for PN we will need a full understanding of the nature of quality through sensory, in-field and chemical measurement and how these attributes vary when novel techniques are trialled, whether in the vineyard or the winery.
The programme starts at the pointy end value chain with very challenging research into understanding what PN “quality” means for both the consumer and the wine expert. At the other extreme end of the PN production process single berries will be grown under tightly controlled environment and nutrition conditions in the lab to develop a deep understanding of factors that influence grape berry composition.
To link these extremes of the programme, work will establish response curves for known key grape constituents (e.g. phenolic composition, aroma precursors) located in or near grape berry skin from intensive sampling and detailed compositional analysis of grapes from controlled experimentation. Expert guided machine learning (Artificial Intelligence) techniques will play a role in integrating results from all aspects of the programme into measurable definitions of quality. Field validation and optimisation experiments in commercial PN vineyard blocks will be used to develop new viticultural and winemaking tools and techniques. Wines from these field trials will then be returned to the expert panel and consumer studies to test the effectiveness of these new tools.
Research Aim 2.5 – Ideotype Vines
Martin D1, Scofield C2, Rutan T1 – Plant & Food Research: 1Marlborough, 2Clyde
This research attempts to objectively describe the appearance and behaviour of an ideal vine for specific Pinot noir (PN) production goals. In particular the study is looking to identify vines within a given vineyard block that have both above average yield and above average fruit quality potential. If these vine types are successfully identified, future work will attempt to understand why certain vines outperform others with the goal of developing management tools to fix underperforming vines.
In 2018 visual selection of 20 unmanipulated PN vines of differing yields and capacities were associated with harvest and berry composition measurements, photography and manual image analysis techniques to assess vine to vine variation in performance characteristics across 12 vineyard sites in three regions. Care was taken to ensure that the genetic material and pruning and training systems were as comparable as possible between vineyards.
Importantly results to date have not shown a relationship between yield and the total soluble solids content (TSS) of the berries (Figure 1). This was true irrespective of whether the yield was expressed in kg per vine or kg per metre of vineyard row length. This lack of relationship applied to the entire sample set across all the vines in all the study sites (N = 220) and equally between vines within every block studied. Yields per vine varied widely within every vineyard in the study irrespective of whether the vine was targeting higher yield and an affordable price point wine, or whether the vineyard was targeting high-priced iconic wines.
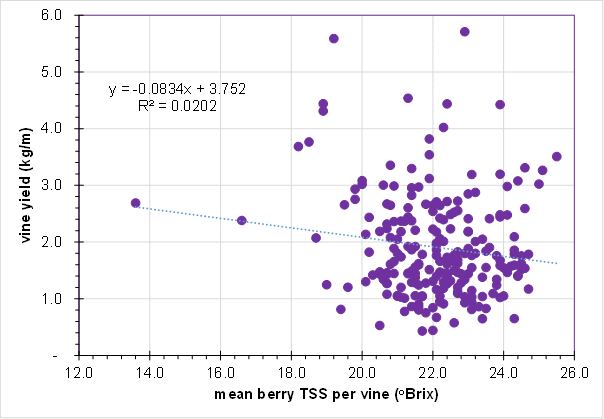
Figure 1. Relationship between mean berry total soluble solids (TSS) and vine yield.
These results are extremely important within the context of the programme because they demonstrate that it is possible to achieve a minimum technological maturity (TSS and titratable acidity) for PN at moderate to high yields per metre of vineyard row length. Across all the vines in the study some 49 (22%) achieved a yield of greater than 1.75 kg per metre of row length and good technological maturity.
Conversely, the majority of vines studied (68%) did not achieve the target metrics, suggesting that factors other than yield were responsible for the under-performance. This result supports the underlying hypothesis of the wider PN Programme, which states that it is possible to break the yield-quality seesaw.
Research Aim 2.6 – Ideotype Wines
Martin D1, Grose C1, Scofield C2, Rutan T1 , Stuart L1, Yang L1– Plant & Food Research: 1Marlborough, 2Clyde
This research attempts to make and analyse Pinot noir wine from single-vine grape lots in order to determine vine and winemaking factors that influence, in particular, wine phenolic composition. A key aspect of our experimental approach is to focus on differences in performance from vine to vine within the same site. This greatly reduces the influence of factors such as region, site and vineyard management on vine performance and subsequent wine composition so we can better understand what other factors might have a predictable and consistent effect on wine quality attributes across a wide production base.
Using a newly designed tube fermenter system we were able to achieve good alignment of key wine attributes between single-vine and commercial block-scale wines thus providing positive signals that the fermenter system was functioning in a repeatable and consistent manner.
The first year of study has affirmed what winemakers have known for a long time: that grapes of very similar soluble solids content can produce wines of widely differing compositions, in particular, their phenolic content. The absence of between-region or between-vineyard effects on 47 of the 50 vine, juice and wine parameters measured supports our experimental approach which has essentially isolated region and vineyard as factors that strongly influence wine composition. Vine leaf area to fruit weight ratio (LA:FW) would appear not to be a key factor influencing differences in juice total soluble solids content or other major wine compositional parameters, especially phenolic composition.
In 2018 there was also a highly significant negative correlation between juice Primary Amino Acid (PAA) and several key individual amino acids, in particular threonine (Figure 2), and wine total anthocyanins.
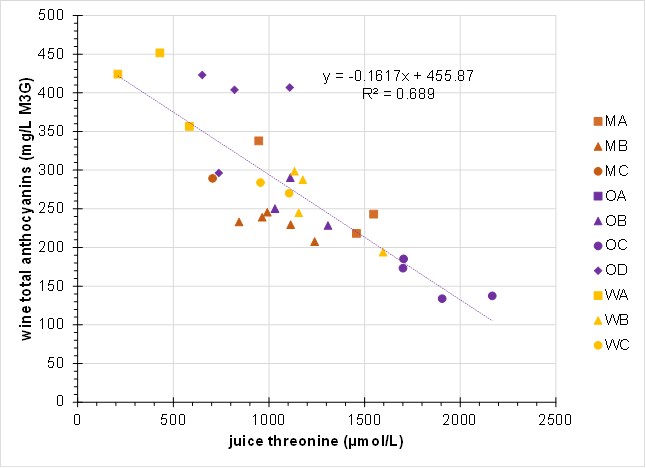
Figure 2. Relationship between cold-soaked juice threonine concentration and wine total anthocyanins, the codes on the right hand side are the vineyard codes.
This result is important to the programme because it suggests that vine nitrogen status could be a major driver of differences in wine colour rather than higher yields or denser grapevine canopies that can indirectly arise. The results also suggest that a trade-off between wine colour potential and wine aromatic potential may occur in PN winemaking.
Research Aim 4.1 – Bunch sorting.
Martin D1, Oliver R2 – Plant & Food Research: 1Marlborough, 2Ruakura
Bunch morphology necessarily affects grape yield on both per vine and per unit area bases. Comparable yields can be comprised of a larger number of relatively small bunches or by a smaller number of larger bunches. In New Zealand Pinot noir (PN) vineyards, the number of bunches per vine within a given vineyard is comparatively stable from year to year, and yield variations are driven more by the number of berries per bunch and/or the average berry mass, which together determine the bunch mass.
The goal of this work is to develop bunch sorting technologies to rapidly and objectively classify PN bunches to determine if larger bunches have the same potential as small bunches to produce high quality wines.
In this study, manual measurements, photography and manual or semi-automated image analysis techniques were used to assess the variation in bunch and berry morphology on grapes from PN Abel Clone grown in Marlborough.
The best bunch image results were achieved with a conventional digital camera above a Compac Sorting InSpectraTM machine vision cabinet (CSIS). The 2D photograph image analysis has, however, limitations in its ability to assess bunch morphology. A general relationship between bunch mass and bunch image area was nevertheless found.
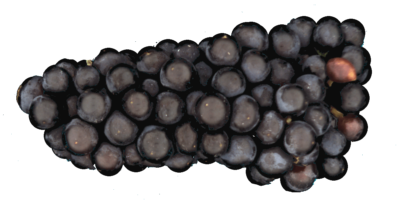
Figure 3. Pinot noir bunch photograph taken on the Compac Sorting InSpectraTM cabinet.
Unexpectedly bigger bunches with high berry numbers did not show a wider distribution of berry sizes than bunches with relatively few berries. This result does however need to be validated in future seasons
Grapes produced from sorting methodologies in RA4.1 will be a resource for RA4.2 – Validation Wines, in which we will produce a series of novel validation wines made from highly characterised grape bunch lots. In 2018 about 300 Pinot noir bunches were sorted for density using a flotation method. A single density class was further sorted into four bunch mass classes of very similar soluble solids content, and fruit were supplied to RA4.2 for replicated winemaking.
Research Aim 4.2 – Validation wines
Grose C1, Stuart L1, Rutan T1, Yang L1, Yvon M1, McLachlan A2 – Plant & Food Research: 1Marlborough, 2Palmerston North
This research continues to build our knowledge on characterising the effects of bunch morphology class and winemaking techniques on secondary metabolite composition of Pinot noir (PN) wine. At Plant & Food Research a series of novel validation wines were made from highly characterised grape bunch lots that were generated from density sorting methodologies in Research Aim 4.1. The novel wines will enable us to better understand the role different bunch morphology classes have on finished wine composition.
During the first year of experimentation we wanted to determine if small-scale research winemaking practices can be used to successfully study the formation and retention of desired secondary metabolites in Pinot noir wine production. Also if making validation wines from sorted bunches of differing mass classes but similar berry soluble solids content have differing wine phenolic composition. The outcomes from this research will determine if we can simultaneously increase both yield and quality using bunch sorting techniques.
Key results showed wine composition from wines made using the newly developed winemaking protocols for the 1.5 and 2 kg tube fermenter system were comparable to the generically accepted parameters for Pinot noir wine production. This is an important outcome for establishing robust, small-scale research winemaking protocols for future implementation within the BRI Pinot noir programme. Bunch morphology class had no effect on juice composition however primary amino acids were less abundant in smaller bunch classes. Wines produced from different bunch mass classes with a similar total soluble solids had similar compositions. However small differences were observed in key wine colour parameters, with colour density 26% higher in wines made from smaller bunches (Figure 4).
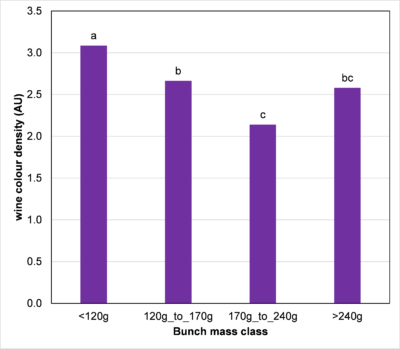
Figure 4. Influence of different bunch mass classes at a similar soluble solids on colour density in Pinot noir wine.
Finished wines were successfully subsampled in to 60ml glass vials using the “Wine in Tubes” system (George Michel Wine Estate, Marlborough) and stored at low temperatures (0‑2⁰C) for further analytical phenolic and volatile chemistry analysis, providing additional important results for the wider BRI PN programme. Results from the first year of research show there may be opportunity to use bunch sorting methodologies to modify secondary metabolite composition of Pinot noir wines and in particular wine colour.
This article first appeared in the February/March 2020 issue of the New Zealand Winegrower Magazine.